AWS Re:Invent 2024: A Data Leader's Summary
Every December, AWS's re:Invent conference brings a flood of announcements that can feel overwhelming, even for technical teams. This year was no different, with over 30 new data-related features announced. As a data leader, you might be wondering what this all means for your organization, beyond the technical specs.
From a strategic perspective, three key themes emerged at AWS Re:Invent 2024
- AWS heavily invested in AI capabilities, launching everything from coding assistants to supercomputers. They launched Amazon Q Developer and Nova foundation models, directly challenging OpenAI's dominance and Anthropic's Claude in the enterprise AI space. (Although they also invested 8 billion in Anthropic). The introduction of multi-agent collaboration in Bedrock also takes aim at Microsoft's Copilot suite.
- They introduced new data governance tools, particularly around metadata management and security controls. With Amazon SageMaker Data, AI Governance and enhanced data lineage features, they are positioning themselves against Databricks' Unity Catalog and pure-play data governance platforms like Collibra.
- And they announced significant improvements to their storage solutions, with S3 Tables bringing Apache Iceberg support and queryable metadata features, competing with Snowflake's native tables and Databricks' Delta Lake.Â
Unsurprisingly, each announcement adds layers of complexity to an already complex landscape. Yet, we believe it should be clear by now (at least to larger organisations) that complexity in the data landscape isn't a problem to solve anymore, we’re way past that point. It’s simply a new reality to embrace.Â
So with every big data industry event like AWS’s annual conference, a more useful approach for the Data Leader is to try to understand how to evaluate, prioritize and adopt what truly adds value. In a nutshell, consider how to effectively manage the added complexity that new tech developments inevitably bring, instead of how to solve it. This can be done by following a structured approach to working with new capabilities - through clear processes for evaluation, adoption, and integration into existing workflows.
The recent SageMaker features, for instance, promise to democratize AI development. But for organisations without clear processes for collaboration and governance, this democratization could lead to chaos. Similarly, AWS's new storage features like S3 Tables offer new capabilities for organizing and querying data. But the technical ability to query data more efficiently doesn't address the organizational challenges of determining who should have access to what data and why.
Evaluate New Tech Announcements Through The Data Product Lens
So while keeping up with new tech updates is important, leaders need a systematic approach to evaluation - one that focuses less on technical details and more on how new capabilities can be effectively integrated into existing organizational processes.
This is where data product thinking comes in. Instead of fighting socio-technical complexity, it provides a framework for operating within it. By viewing data as a product with clear ownership, defined interfaces, and standardized processes, organizations can navigate complexity confidently. Â
So whether you're evaluating AWS's latest data/AI announcements or considering any new data technology, you can use this perspective to cut through the noise. It makes it easier to understand what may be an opportunity and what may be a hindrance. For organisations that are already managing large data systems and are well aware of the drawbacks of centralised data architectures, evaluating new capabilities through the data product lens can mean asking things like: How does this new capability fit into our existing data product architecture? Does it help standardize how teams deliver and consume data? Can it strengthen our data product interfaces and contracts?
For example, AWS's new S3 Tables feature could strengthen data product interfaces by providing standardized ways to expose and consume data. Their enhanced governance features could help automate data product quality measurements and access controls.Â
Â
Â
AWS Re:Invent 2024: The Data Product Approach
So here’s how we can look at AWS's announcements through specific data product considerations.
Does any of this help data product teams deliver value faster?
The new AI capabilities in Amazon SageMaker, like HyperPod flexible training plans, promise faster model development through optimized compute resources. This speed advantage materializes if your organization has clear processes for model deployment and validation. Without them, faster development could actually lead to more technical debt and governance challenges.
Will any of this make governance models more efficient?
Amazon SageMaker's Data and AI Governance features offer automated policy enforcement and improved data lineage. These require significant setup and maintenance. Organizations with well-defined data product ownership models will find these features amplify their existing governance processes. Those still struggling with basic questions like "who owns this data?" might find these tools add another layer of complexity without solving fundamental challenges.
Can any of this improve collaboration between data product owners and consumers?
The enhanced storage features, like S3 Tables, could either streamline or complicate collaboration, depending on your organization's maturity. While they enable better data organization and querying, they also introduce new concepts and workflows that teams need to understand. Success depends less on the technical capabilities and more on having clear processes for how teams should work together.
These questions help shift the focus from technical capabilities to organizational readiness and process maturity. This is the approach we take at Dataminded when delivering strategic data consultancy and also with Portal, a new open-source data product management tool that provides a guided process for creating data products and that enables governance by design for data initiatives.
Conclusion
As with any new announcement, there's excitement around the AWS releases for opportunities to get more out of our data initiatives. The challenge lies in how we approach these advances. By viewing them through the lens of data product thinking and process, we can better prioritize and focus on standardized approaches that help teams collaborate effectively and deliver value consistently.
TL;DR
For leaders that embrace the decentralized governed approach of data products, several announcements made at AWS re:Invent 2024 show promising alignment with data product thinking.
- Amazon SageMaker's Data and AI Governance features could automate quality measurements for data products
- S3 Tables' support for Apache Iceberg could make data product interfaces more standardized and interoperable
- Enhanced data lineage features could help track relationships between data products
These developments suggest the industry is moving toward more structured approaches to data management, though implementation success will still depend on clear organizational processes.
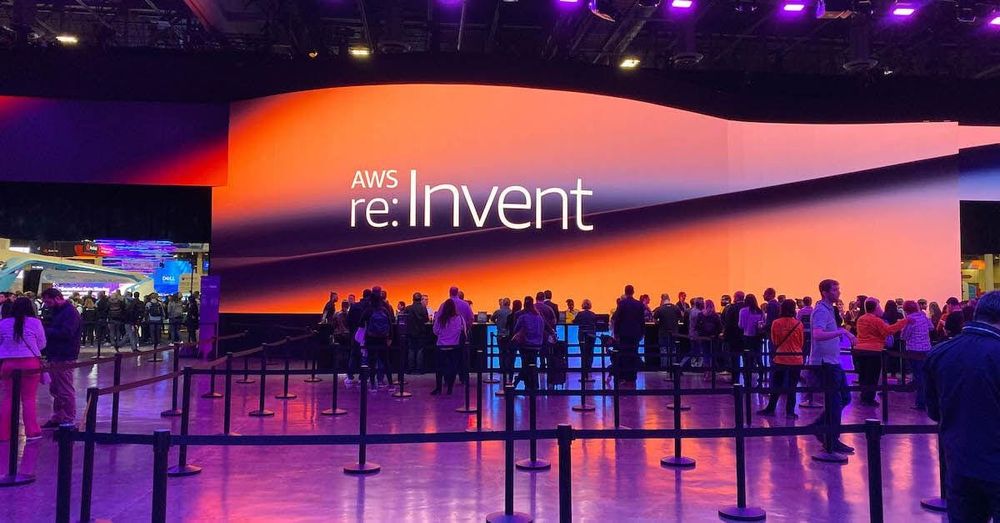